Key Difference between Generative AI vs Predictive AI
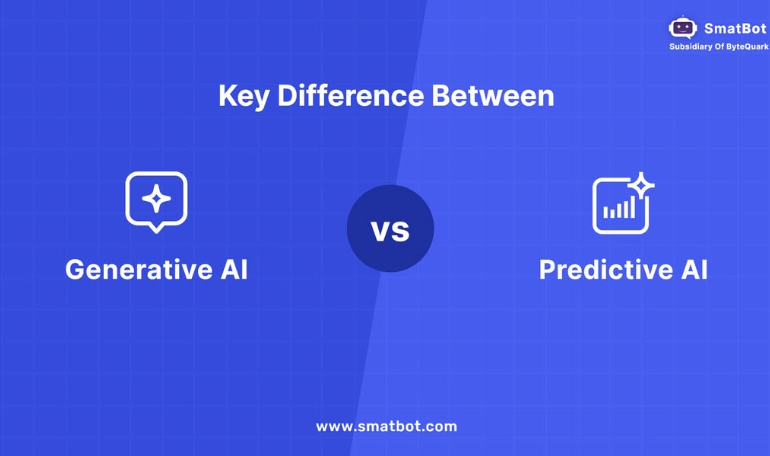
Key Difference between Generative AI vs Predictive AI
Imagine AI generating a logo that perfectly captures your brand essence or predicting what product a customer will buy next. This is the power of generative AI and predictive AI.
As two standout technologies in the digital era, understanding which AI tool is right for your business is crucial. We’ll compare both technologies, discuss their applications, limitations and benefits and also share some real-life examples.
By the end of this article, you’ll surely know which AI model suits your business best.
So, without further ado, let’s get started.
What is Generative AI?
Generative AI leverages advanced algorithms and neural networks to learn from large datasets and generate creative content like music, audio, images and text. For example, it can generate artwork resembling famous painters’s styles.
Moreover, Generative AI can be used to compose original music and design realistic images for video games. Not only this, but it can also help in creative writing. Its knack for generating novel content unlocks new opportunities for human creativity and expression.
However, developing generative AI models involves collaboration across various disciplines including research, machine learning operations, programming and user experience. This multidisciplinary approach ensures that these models are created, trained, deployed and maintained ethically and responsibly.
Limitations of Generative AI
- Training state-of-the-art generative models requires a lot of computing power. It emits substantial carbon, necessitating environmental responsibility.
- AI-generated deep fakes and manipulated media carry risks like spreading misinformation and social manipulation.
- Generative AI can struggle with long passages. It’s sensitive to wording changes and can produce inconsistent responses.
- Sometimes the generated data may lack factual accuracy or overall coherence.
- If you provide incorrect or incomplete prompts then it might generate insensible data.
Benefits of Generative AI
- Enhanced efficiency and productivity
- Realistic simulations
- Enhanced creativity
- Time and cost savings
- Improve customer experience
- Data synthesis
- Hyper-personalization
Examples of Generative AI Applications:
- Video Creation
Synthesia is a real-life example of Generative AI which is an AI video generator. It can create eye-catching videos from text prompts. The best part is that it generates video with human-like presenters which are also called AI avatars. This tool helps online educators to transform their documents into compelling videos.
- Ad Creatives and Social Media Posts
AdCreative.ai is another excellent generative AI tool that generates ad creatives and engaging social media posts. It can specify the right platform, target audience and select the ideal message for your specific business goals.
- Image Creation
Midjourney is an AI image generator that turns text prompts into realistic images. It pushes the boundaries of visual art creation by generating intricate compositions, realistic edits and diverse details.
- Chatbot Building Solution
At SmatBot, we offer advanced AI chatbot building solutions that make creating engaging conversational experiences a breeze. With our intuitive interface and no-code chatbot builder, you can easily train your bot on relevant data and design conversations that perfectly match your business needs.
Whether you want to capture leads, allow customers to book appointments, provide stellar customer support or automate repetitive tasks, SmatBot offers a seamless solution to boost engagement and make your business operations run smoother.
Additionally, we offer numerous third-party integrations. These integrations allow you to connect your chatbot to your existing business tools and apps.
- Coding
Generative AI can automate the code creation process, making coding accessible to non-technical users. It offers features like code suggestions and bug identification, ensuring adherence to coding guidelines for readability and consistency. OpenAI and Codex are some of the best examples.
What is Predictive AI?
According to a report by Rivery, humans generate about 2.5 quintillion bytes of data per day. Businesses employ predictive analytics to streamline operations and increase profits by generating insights and forecasting future outcomes from large datasets.
Predictive AI is a branch of predictive analytics that uses machine learning and statistical algorithms to analyze large datasets and forecast trends, behaviours and patterns.
Additionally, this AI model helps businesses predict future outcomes and improve their decision-making. Many industries like supply chain management, manufacturing and marketing are using Predictive AI to enhance their efficiency and strategies.
Limitations of Predictive AI
- The accuracy of results depends on the quality of the training data. Biased or insufficient data can lead to inaccurate predictions.
- These models require a lot of resources for training and deployment which can make them impractical in some situations.
- Complex predictive models such as deep neural networks are often hard to interpret. It can be tough to figure out how the AI makes predictions or detect any biases in its decisions.
- It’s limited because it relies on data that already exists and can’t predict unexpected events.
Benefits of Predictive AI
- Improved Decision Making and Forecasting
- Personalized Experiences
- Real-Time Analysis
- Fraud Detection
- Cost Savings
- Competitive Advantage
Examples of Predictive AI Applications
- Fraud Detection
Cybersecurity is a big concern today and predictive analytics plays a crucial role, especially in fraud detection. It scans for unusual activity in systems and flags potential threats. Predictive AI is crucial for stopping hackers and preventing system breaches. It uses past cyber attack data to spot similar threats and alert the right person.
Examples include Banks and financial institutions.
- Content or Product Recommendation
Content or product recommendation systems powered by predictive analytics are widely used in the e-commerce and entertainment industries. By analyzing user watch history or past purchases, these systems predict what users are likely to enjoy or purchase.
For example, Netflix uses predictive analytics to suggest content based on user preferences such as genre and search history. Similarly, online shopping sites like Myntra use predictive analytics to recommend products based on past purchases.
- Up-Selling and Cross-Selling
Up-selling and cross-selling aim to increase revenue from the existing customer base. By analyzing purchase history data, predictive AI suggests complementary products or services to existing customers to increase customer revenue. This boosts sales for your business while providing customers with items that complement their purchases.
Examples include Banking, Retail, Insurance, and E-commerce
- Inventory Management
Predictive AI helps in inventory management to predict product demand based on factors like location, season and buying patterns. This helps retailers maintain optimal inventory levels, preventing overstocking or shortages. PepsiCo is one of its best examples which has freed up around 4,300 workdays per year by automating routine tasks and focusing more on strategic activities.
- Healthcare Predictive Analytics
SmartBot utilizes advanced algorithms and machine learning to analyze extensive patient data, comprising medical history, diagnostic tests, genetic information, and more. Through this analysis, SmatBot predicts patient outcomes, disease progression, and the probability of adverse events. These invaluable insights enable healthcare providers to make informed treatment decisions, leading to enhanced patient care and improved outcomes.
Key Difference between Generative AI vs Predictive AI
Generative AI and predictive AI models differ in several ways. Understanding these differences can assist in selecting the appropriate model for your specific business needs.
Factors | Generative AI | Predictive AI |
Purpose & Goals | The goal of Generative AI is to produce content that mirrors the style and structure of its training data. It aims for output indistinguishable from human-created content. It works across various domains including literature, art, music and scientific research. | Predictive AI is all about predicting future outcomes based on historical data. Its goal is to provide reliable and accurate predictions that can help in decision-making. It plays a crucial role in areas where precise predictions can save time and resources and prevent damage, such as finance, healthcare, weather forecasting, marketing, and more. |
Algorithmic Structures | Generative AI employs advanced technologies like Variational Autoencoders and Generative Adversarial Networks (GANs). Variational Autoencoders encode, modify and then decode data to generate new variations. Generative Adversarial Networks involve two neural networks working together to create realistic and new data. These techniques allow Generative AI to make a wide range of creative content, opening up lots of new possibilities for creativity. | Predictive AI using several technologies such as classification algorithms, neural networks, regression analysis and decision trees. Classification algorithms categorize data into different groups. While regression analysis forecasts continuous outcomes. Decision trees analyze data by following different paths to reach conclusions, while neural networks imitate how the human brain works to recognize patterns and make predictions. When used together, these technologies help Predictive AI study past information and forecast future trends accurately. |
Complexity Level | Generative AI is quite complex and needs computational power and advanced algorithms. Learning patterns and creating realistic outputs takes a lot of computing power. Techniques like GANs and VAEs are challenging and require advanced algorithmic understanding and strong hardware. | Predictive AI complexity varies by its use. Simple models like linear regression suit basic predictions in stable markets such as sales forecasts. Advanced models like deep neural networks, handle complex data with many variables like predicting financial trends or consumer behavior. |
Output Objectives | Generative AI aims to create something new, not just replicate what’s been seen before. For example, it’s like a tool for graphic designers who want fresh logo ideas. Instead of starting from scratch, they can use generative AI to produce innovative designs based on its training. Authors can use it to brainstorm unique plot points and musicians can use it to create new melodies. | Predictive AI looks at past data to predict future events accurately. It’s like how meteorologists use historical weather data to forecast the weather. They’re not inventing new weather patterns but making informed predictions based on past patterns. |
Applications | Generative AI is changing how we create in design, art and music, sparking creativity across different fields. It empowers artists to create unique artwork and original music compositions while streamlining content creation by generating text, images and videos. In addition, Generative AI designs and populates virtual worlds for video games, simulations and virtual reality. Additionally, it helps in the fashion and product design industry by creating new designs and predicting trends. | Predictive AI finds diverse applications across industries. It forecasts stock market trends, investment opportunities and financial risks by analyzing historical financial data. Businesses use it to analyze customer data, predict purchasing patterns and tailor marketing strategies accordingly. In healthcare, it aids in disease diagnosis and treatment planning. Moreover, it helps in fraud detection and identifying fraudulent activities to prevent financial losses and cyber threats. |
Use cases | Generative AI is used for various creative tasks such as data augmentation, content creation and code generation. | Predictive AI is used for financial forecasting, business analytics and decision support. |
Conclusion :
And that’s a wrap! We’ve covered almost every aspect of generative AI and predictive AI in this comparison guide.
By understanding their key differences, we can see how these AI models are not just transforming industries, but pushing the boundaries of what AI can achieve.
The future holds even more exciting possibilities as Generative AI fuels creativity and Predictive AI helps us navigate an increasingly complex world.
We hope this article helped you understand the difference between generative AI and predictive AI and why they’re important for humanity and AI advancement.
Considering leveraging Generative AI for your business operations? Our platform leverages generative AI in various applications to enhance language understanding and document summarisation capabilities. With GPT integrated into websites, databases, and personal knowledge base documents, users can ask natural language queries and receive accurate responses or summaries tailored to their needs. This empowers businesses to streamline information retrieval, facilitate natural language interactions, and generate concise summaries of lengthy documents for efficient decision-making.
So, why wait? Book a demo with us today, our experts will assist you in your journey towards the generative AI world.